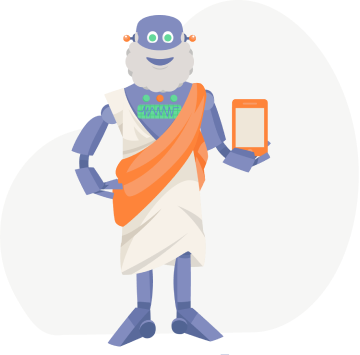
Why?
This lesson focuses on the intersection of AI and music, exploring how algorithms curate personalized playlists and the implications for musical diversity and artist exposure. It raises critical discussions on the challenges of algorithmic bias and echo chambers, alongside ethical considerations like privacy and copyright in the digital music landscape. By engaging in simulations and reflective discussions, students will understand the complexities of AI-driven music recommendations and consider how technology can better serve both listeners and artists in a diverse musical ecosystem.
Materials Needed
Printouts of the simulation sheet for each group: 1 Playlist Rules Group sheet Enough copies of the Song Suggest sheet for all other groups Document camera (optional) to display suggested song characteristics
Time needed
45 - 60 Mins
Objectives
- Students will be able to explain how AI algorithms influence music playlist recommendations and the concept of an “echo chamber.”
- Students will be able to identify the challenges and limitations of AI in promoting musical diversity and supporting emerging artists.
- Students will be able to discuss ethical considerations in AI-driven music recommendations, including privacy concerns and copyright issues.
- Students will be able to propose potential improvements or solutions to enhance the fairness and diversity of AI-driven music recommendations.
Key Concepts & Vocabulary
- Echo Chamber: A situation where AI repeatedly recommends music similar to what a listener has previously enjoyed, potentially limiting exposure to new genres or artists.
- Algorithmic Bias: The tendency of AI algorithms to reflect or amplify biases present in their training data or design, possibly favoring certain types of music over others.
- Playlist Curation: The process of selecting and organizing songs into a playlist, which, in the context of streaming services, is often influenced by AI to match listeners’ preferences.
Lesson Components
- Before You Watch: Connect lesson to background knowledge of AI algorithms in music playlists and get students’ attention.
- Video: Show the pedagogy.cloud video explaining the ethical considerations in the topic of algorithmic recommendations.
- Case Study: Detail a real-world scenario that relates to the issue of AI in playlist algorithms.
- Simulation: Lead students through an interactive activity exploring the possible ethical considerations.
- Discussion: Ask whole-class questions to reflect on experience and consider perspectives.
- Assessment: Verify student understanding with an exit ticket.
Warm Up
Option A: Music Genre Brainstorm: Have students quickly list as many music genres as they can in two minutes, highlighting the diversity in music and setting the stage for discussing how AI manages this diversity.
Option B: Personal Playlist Sharing: Invite students to share one song from their favorite playlist and explain why it’s there, introducing the concept of personalized recommendations.
Video
Transcript
Hello Young Innovators! Today we’re discussing the ethics of gendered voices of AI assistants.
Artificial Intelligence is becoming a bigger part of our lives every day. From smartphones to smart homes, AI voice assistants are everywhere, helping us with tasks, answering our questions, and even keeping us company. But have you ever wondered why most of these voice assistants sound female?
AI voice assistants haven't always been around. In the early days of technology, computers were large, clunky machines that certainly didn’t talk. As technology evolved, so did the ability for machines to interact with us using voice – a feature that is becoming increasingly common.
Imagine asking your AI for the weather, and a deep, authoritative voice responds. Or, picture a soft, gentle voice helping you with homework. Why do these differences matter? Well, they bring us to our main topic: the ethics of gender representation in AI voice assistants. For a long time, most AI assistants like Siri or Alexa had female-sounding voices. This wasn’t just a random choice.
Research showed that people generally found female voices to be warmer and more welcoming. And people were used to hearing women’s voices from back when operators connected phone calls.
On the flip side, some people prefer to hear male voices for authoritative roles, like GPS navigation or voiceovers in documentaries. But this leads to ethical concerns. Are we reinforcing traditional stereotypes about gender roles, stereotyping men in roles of power and women in roles of service?
One method of dealing with this issue is to use gender-neutral voices. These are designed to not clearly sound male or female, aiming to represent a wider range of human experiences and identities. It's a step towards inclusivity, and an attempt to avoid the stereotypes of gender from previous generations.
When AI voice assistants reinforce gender stereotypes, they might also impact how we view gender roles in real life. But when we make these voices gender-neutral, are we erasing gender differences that are a real part of many people's identities?
Some people argue that having a range of gendered voices in AI can reflect the diversity of human experiences. Others believe that breaking away from gendered voices entirely is the key to challenging stereotypes and promoting equality. There’s no easy answer, and technology is constantly evolving to reflect our changing society.
So, what do you think? Should AI voice assistants have a gender? Or should they be gender-neutral to avoid reinforcing stereotypes? As we continue to integrate AI into our daily lives, it's important to think about how the choices we make about technology today shape our future.
Let’s discuss: How do AI assistants impact our attitudes toward gender in the real world?
Case Study
Distribute or read Case Study handout.
Summary: Leon Harmonia, a musician blending jazz and electronic music, receives local acclaim but struggles to gain global recognition on streaming platforms due to AI algorithms favoring mainstream genres, creating an “echo chamber” effect. This challenge is compounded by concerns over privacy and copyright with AI’s use of song snippets for recommendations. Addressing these issues requires enhancing AI for greater musical diversity, introducing user-driven discovery features, ensuring transparent data practices, and providing artist support programs to balance personalization with creative diversity.
Student Handout
Case Study: The Frustrated Musician
A talented musician, known as Leon Harmonia, with his unique blend of jazz and electronic music, gets rave reviews at local venues whenever he plays. But he has remained virtually unknown on the global stage, despite being available on all major streaming services.
Leon Harmonia is celebrated in his local community for his captivating live performances that seamlessly merge jazz with electronic beats, creating a sound that’s both nostalgic and futuristic. Eager to share his music with the world, Leon uploaded his tracks to various streaming platforms, expecting new listeners to discover his innovative tunes.
However, Leon encountered a perplexing challenge: his music wasn’t reaching new audiences as he had hoped. AI playlist algorithms, designed to suggest songs based on users’ previous listening habits, tended to favor more mainstream genres, leaving niche and genre-blending artists like Leon in the shadows. This “echo chamber” effect limited the diversity of recommendations, stifling the discovery of fresh, unconventional sounds.
Furthermore, Leon and other artists in similar situations voiced concerns about privacy, as AI collected intricate details about listeners’ preferences, and about copyright, given the platform’s use of song snippets for generating recommendations, sometimes without clear artist authorization.
Possible Solutions
Algorithmic Diversity: Enhancing AI to value a broader spectrum of music, ensuring that innovative and less conventional genres are also recommended to listeners.
User-Driven Discovery: Introducing features that allow users to explore music outside their usual preferences, such as a “Discovery Mode,” which could spotlight underrepresented artists and genres.
Transparent Practices: Streaming services could adopt data practices that clearly explain how data is used and ensure all recommendations comply with copyright.
Artist Support Programs: Establishing initiatives to support artists like Leon in navigating the digital landscape, offering guidance on how to increase their visibility on streaming platforms.
Conclusion
The tale of Leon Harmonia sheds light on the double-edged sword of AI in music streaming: while offering unprecedented personalization, it also risks overshadowing the rich variety of diverse musical expressions. Addressing this dilemma requires a concerted effort to balance technological innovation with the nurturing of creative diversity, ensuring every artist has the chance to be heard on the global stage.
Reflective Questions:
- How would you feel if your music or a genre you’re passionate about was overlooked by streaming platforms’ recommendation systems?
- What other strategies could help ensure that artists like LeonHarmonia find their audience in the digital music ecosystem?
Simulation
The classroom is transformed into a music curation lab, where students are divided into small groups, tasked with either suggesting songs for a playlist or setting the rules for song inclusion based on various characteristics.
Divide the class into small groups of about 3-4.
Roles
- Playlist Rules Group: One group randomly chosen to set the criteria for songs to be included in the playlist. They decide on desired characteristics like tempo, mood, genre, and vocalist gender.
- Song Suggest Groups: Remaining groups work as music curators, suggesting 5-6 existing songs each, and listing their characteristics based on the provided criteria.
Tasks:
- Setting Up: Distribute a characteristic sheet to all groups, outlining categories like tempo, mood, genre, and vocalist gender.
- Rule Creation: The Playlist Rules Group discusses and decides on specific characteristics they seek in playlist songs.
- Song Suggestion: Song Suggest Groups brainstorm and list 5-6 songs with their corresponding characteristics, ensuring a mix of genres and styles.
- Presentation: Teacher displays the characteristics of suggested songs without revealing titles, maintaining anonymity.
- If you have a document camera, you can put each group’s list on the screen one at a time, making sure to fold the left side of the page over to cover the song titles.
- If not, you can have each group read the song characteristics, and have the Rules group pick by letter after listening to the lists.
- Selection Process: The Playlist Rules Group reviews the song characteristics and selects one song from each Song Suggest Group that best fits their criteria, noting the group number and song letter.
- Reveal and Reflection: Once selections are made, song titles are revealed, and the class reviews the playlist to discuss the diversity and similarity of the chosen songs.
- (Optional) Listening Time: songs could be played in class to see how closely they fit the playlist. (Remembering. of course, that not all suggested songs will be appropriate for school.)
- Bring the class back together for whole-class discussion on questions listed in the next section.
Student handout
Simulation Activity: Playlist Curation Challenge
Playlist Rules group: In the top row, list the characteristics you want to be a part of this playlist. Do not share those characteristics with other groups. When the other groups have listed their songs, your role is to pick the one song from each group that best fits your rules, and add its letter to your playlist. Titles will be revealed later.
The Rules
Tempo
(Slow, Medium, Fast) |
Mood
(Upbeat, Sad, Relaxing, Aggressive, etc.) |
Genre
(Pop, Rock, Hip Hop, Folk, Country, etc.) |
Vocalist
(Male, Female, Group, Instrumental) |
The Playlist
Group # | Song Letter | Title |
1 | ||
2 | ||
3 | ||
4 | ||
5 | ||
6 | ||
7 | ||
8 | ||
9 | ||
10 | ||
11 | ||
12 |
When song titles are revealed and discussions ensue, respect your peers’ choices and opinions, fostering a constructive and inclusive environment.
Simulation Activity: Playlist Curation Challenge
Song Select groups: Come up with a list of 5-6 songs to suggest for the playlist. Your goal is to create a diverse and engaging selection that showcases a wide range of musical expressions, aiming to challenge and expand the playlist criteria set by the Playlist Rules group.
Group #: _______
The Songs
Song | Song Title | Tempo
(Slow, Medium, Fast) |
Mood
(Upbeat, Sad, Relaxing, Aggressive, etc.) |
Genre
(Pop, Rock, Hip Hop, Folk, Country, etc.) |
Vocalist
(Male, Female, Group, Instrumental) |
A | |||||
B | |||||
C | |||||
D | |||||
E | |||||
F |
When song titles are revealed and discussions ensue, respect your peers’ choices and opinions, fostering a constructive and inclusive environment.
Discussion
These questions are designed to be used in whole-class discussion. Ask questions that relate most effectively to the lesson.
- How did the role you played in the simulation affect your perspective on how music playlists are curated?
- In what ways did the activity challenge your understanding of AI’s impact on music discovery?
- Did this simulation change your thoughts on the diversity of music available on streaming platforms? How so?
- How did the criteria set by the Playlist Rules group influence the variety of songs selected by the Song Select groups?
- Were there any surprises in the songs, or types of songs, that were selected or overlooked? What does this reveal about AI-driven recommendations?
- How might the limitations of AI in recognizing diverse musical genres and styles impact emerging artists
- How do you think AI algorithms could be improved to better support musical diversity and discovery?
- Considering the simulation, what role do you think listener feedback should play in shaping AI music recommendations?
Assessment
Exit Ticket: Provide a prompt for students to reflect on their learning, such as:
- What is one new insight you gained about AI and music playlist curation from today’s lesson?
- How do you think AI-driven music recommendations can be improved to support a wider range of artists and genres?
- Reflecting on the simulation, what is one challenge you think AI faces in accurately reflecting musical diversity?
Sources to Learn More
- Is Spotify’s algorithm helping or hurting musicians? – https://amt-lab.org/blog/2022/4/spotifys-algorithm-helping-or-hurting
- Background of Spotify’s “Daily Mix” algorithmic playlists – https://medium.com/systems-ai/spotifys-machine-learning-algorithms-and-your-daily-mix-f49d97db4b16
- What role do humans play in AI algorithms? https://www.complex.com/pigeons-and-planes/a/eda-yu/youtube-spotify-editorial-playlists-algorithm-human-connection
- A picture of how Spotify’s algorithm works – https://www.makeuseof.com/decoding-how-spotify-recommends-music-to-users/
- Article about why Spotify seems to play the same songs over and over – https://apps.uk/why-does-spotify-play-the-same-songs/